Using Predictive Analytics To Discover And Protect Sensitive Enterprise Data
How AI Is Used For Data Risk Management
Add bookmark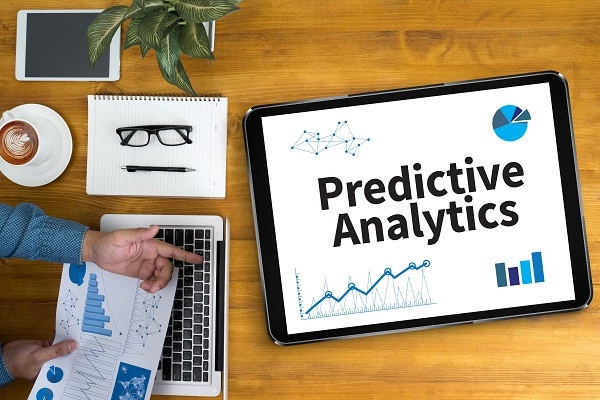
Data is creating new value for enterprise organizations. Historical sales information is used to predict prospective clients and repeat customers. Reporting is increasingly automated and customized to the needs of the manager. And knowledge workers are empowered with data to make informed business decisions. But at what cost does this digital landgrab potentially push employees beyond their limits and create new, unforeseen risks for the business?
Consider industry darling, Enron, named annually by a leading business publication as its “Most Innovative Company in America” from 1996 to 2001 and eventually became one of the largest bankruptcies in corporate history. In retrospect, the writing was on the wall for the organization. Internal communications indicated a pattern of employee discrimination and squelching whistleblowers who flagged questionable or illegal company practices. What legal teams lacked at the turn of the century was the ability to analyze the disparate data sources — internal memos, email, servers — to identify the patterns in data as they were developing.
The stark reality is that the number of company lawsuits continues to grow. According to data from the U.S. Courts, more than 3,000 patent cases were filed in 2018. The cost of business litigation is also increasing per incident. An undeterminable amount of loss also occurs from innovation and intellectual property development that is not captured by the organization, whether it be due to a lack of awareness or the inability to develop a process encouraging employee participation.
With more information being generated within the enterprise at exponential rates, the data is quickly growing beyond the human capability to analyze it. Simply hiring more auditors and business analysts is not possible for most organizations.
See Related: How To Properly Define A Threat Hunting Operation
Enter AI For Risk Management
Organizations have a lot of sensitive data — from privileged information to personal information and HR topics from discrimination to sexual harassment. Data privacy legislation — from GDPR to CCPA — is giving enterprises cause to track information’s origin and where it resides in personal data.
One method being pursued by corporate counsels and external legal teams is the use of machine learning and deep learning to uncover sensitive data hidden within enterprise data. Unstructured data from disparate sources is combined with natural language processing (NLP) to match language with identified risk factors. Ultimately, this process of automation leads to increased electronic discovery (eDiscovery) for the organization.
“AI is both a risk and a way to manage risk,” says EY’s Global Assurance Innovation Leader Jeanne Boillet.
These intelligent systems are not fool-proof and “false positive” indicators do occur. And this is where human intelligence still outpaces machine intelligence due to the complex and subjective nature of a business’s operations. A relevancy indicator escalates review by management and a determination can be made to pursue the situation further or not.
Enterprise leaders and advisory boards need to ask themselves, “How is the organization using AI technology and new data sets for governance and risk management?” adds Boillet. The value to an industry and enterprise business can take many shapes. Some of the desired outcomes from this automation approach include helping enterprises:
- Uncover the movement of sensitive company data
- Identify at-risk employees based on communications patterns
- Determine which workers have habits that lead to innovation and intellectual property development
- Monitor compliance in regulated industries
- Reduce enterprise-wide risk and manage corporate reputation
- Mitigate legal, compliance, and privacy risks
See Related: The Economic Side Of Cyber Security Risk Management
Assessing The Risk Of AI For Enterprise Data Risk Management
The Association of Certified E-Discovery Specialists (ACEDS) reports that sanctions are increasing for companies that do not turn over electronic data. In August 2016, an airline was ordered to pay $2.7 million in sanctions for failing to follow the eDiscovery process. This was in addition to another $4.7 million in sanctions that had already been ordered for discovery violations.
The AI approach to risk management of enterprise data helps companies find the needle in the haystack themselves while keeping liability within the organization. Empowering people to discover and assess the risk remains the focus over displacing workers with intelligent systems that are only as good as the training datasets used.
Each industry and business needs to define things in legal and privacy matters. Approaches need to offer a baseline functional capability, plus options to customize for an organization’s regulatory domain and company culture. Not only what counts as personal information today, but adaptability as the system learns new patterns, and potential areas of risk. Identifying more locations for sensitive data and the recovery of work data residing on devices outside of company-operated platforms is a growing concern. The AI-powered approach also has challenges, such as text summarization, where no definition exists. However, there are objective rules that allow for forward movement and progress to occur.
Organizations looking to build confidence in automating data risk management should consider a trial that places the AI approach in parallel to status quo methods for identifying sensitive information. Only then can you determine if the technology (and your business) aide in the risk management of data.
This data risk management article originally appeared on our sister site, Enterprise Digitalization.
See Related: Risk Of Data Loss More Than A Security Problem; It’s A Business Problem