The 4 stages of data lifecycle management (DLM)
Add bookmark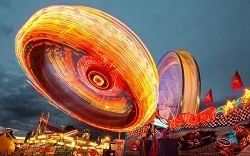
In an era dominated by digital connectivity, safeguarding the integrity and security of organizational data is vital. At the core of robust data security practices lies data lifecycle management, an organizational framework that guides the trajectory of data from its inception to ultimate disposal.
Read on to find out about the various stages of DLM, including data creation, storage, usage and archival, with a particular emphasis on strategies to fortify data security.
What is data lifecycle management?
A central component of data governance is data lifecycle management (DLM) - the organizational processes used to control data from its creation to destruction. The goals of DLM are to:
- Ensure regulatory compliance. DLM ensures your company’s data practices are compliant with both local and international laws such as GDPR.
- Enhance governance. By making a repeatable and understandable DLM process, you are improving organizational governance and IT governance.
- Data loss prevention. Because DLM defines how data is processed, stored, and shared, it can establish practices that prevent loss
- Enable process optimization. With a strong DLM strategy, data is easily accessible, clean, and usable. This means processes can be more efficient and more easily automated.
How is DLM different from ILM?
DLM differs from information lifecycle management (ILM) in that it is less focused on the value of the information. ILM, on the other hand, seeks to understand how the value of data changes over time and how that value can be maximized throughout its lifecycle.
What are the different stages of data lifecycle management?
-
Data creation
Organizations generate vast amounts of data every second of every day. This data can include anything from transactional sales data to website clicks to PDFs. Generally speaking, data is created in 3 different ways:
- Data acquisition: acquiring already existing data which has been produced outside the organisation
- Data entry: manual self-service entry of new data by personnel within the organization
- Data capture: data generated by devices used in various processes in the organization
At this stage, organizations are especially vulnerable to “dirty data” - the collection of inaccurate, incomplete, inconsistent, or duplicate data. “Dirty data” is often the result of manual error but can be caused by more malicious factors such as data poisoning.
The DLM strategy should define the data types used across the business, as well as where they’re used, what they’re used for, and who can use them. Checks should be in place to ensure that data (structured or unstructured) is reliable and complete at the point of origin.
-
Storage
Data must be stored in a way that is both secure but easily accessible by those who need it. At this stage, it is also important to establish data backup and recovery plans to minimize downtime in the event of systems failure or breach.
-
Usage and sharing
An effective DLM strategy will define who can access what types of data as well as how it can be used. This involves ensuring that data flows seamlessly between various systems, dashboards and analytics tools. Encryption and other data obfuscation methods are often leveraged to ensure data confidentiality.
Part of this process is also ensuring that data is not shared via “unofficial means.” This requires the implementation of clear-cut data sharing guidelines and approved data sharing tools.
-
Archival and destruction
Once data is no longer active or of use, it must be safely, securely and lawfully disposed of. The first step in the process is often archiving - the copying of data to an environment where it is stored in case it is needed again in an active production environment, and the removal of this data from all active production environments.
However, data cannot be archived forever as the cost and regulatory risk of storing data only increases over time. Data must eventually be destroyed but doing so can be more difficult than expected.
A company’s DLM strategy must include a detailed standards outline when data should be permanently deleted and how. For sensitive data, secure deletion methods -whereby the original data on that device becomes inaccessible and cannot be reconstructed - are required.
Those who fail to properly control and oversee data deletion practices face tough penalties. For example, Morgan Stanley was fined $60 mn by the OCC after failing to decommission two Wealth Management business data centers. Though the bank hired a third-party vendor to wipe data from servers and other hardware, some customer information remained on the equipment after it was sold to a recycler, resulting in exposure.
In addition, many employees store sensitive data on their personal devices. Once these devices are disposed of and out of the control of the company, it becomes incredibly vulnerable to risk. This is why data destruction strategies must encompass IT asset disposal as well.
Get the latest insights on the cyber threat landscape
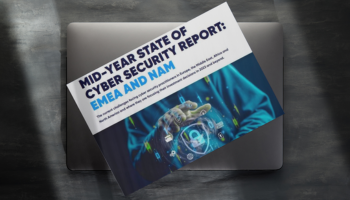
Download our 'Mid-Year State of Cyber Security Report' to learn about the current challenges that cyber security practitioners in Europe, the Middle East, Africa, and North America are facing, and discover where they are focusing their investment decisions in 2023 and beyond.
Read More