Data Auditing: Creating Security Conclusions About Data Set Properties
Add bookmark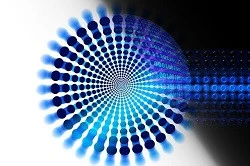
Data quality, or lack thereof, is a growing crisis for many entities. Not only does poor data quality hinder decision-making, it can result in substantial compliance, reputational and financial risk. To start, the average company loses 12% of its revenue per year due to data quality issues. Furthermore, nearly one third of analysts spend more than 40% of their time vetting and validating their analytics data before it can be used for strategic decision-making.
In order to truly capitalize on the next wave of technology with the like of artificial intelligence and machine learning, organizations must optimize the quality and usability of their data while also ensuring it is protected against potential threats.
A data audit refers to assessment of data quality to ensure its accuracy and efficacy for specific usage throughout its lifecycle. This involves profiling the data in question and assessing the impact of poor quality data on the organization's performance and profits. Auditing data, unlike auditing finances, involves looking at key metrics, other than quantity, to create conclusions about the properties of a data set.
To elaborate, some of the key areas data auditing examines are:
- Data accuracy – validating data for integrity, completion and classification.
- Regulatory compliance – ensuring data and processes adhering to corporate, industry, and government regulations when it comes to location, storage, usage, and data security.
- Data sources – the process of “qualifying” external data sources to ensure they deliver high integrity data.
- Data volume & scale – looks at what data is being collected, where/how is it being stored.
- Isolated, duplicated & anomalous data.
- Data flows and systems – are data-related systems performing as intended and delivering optimal outputs.
- Data protection & security – ensuring data is safeguarded throughout its lifecycle.
- Data ownership – who is accountable for managing and using data.